Data Wrangling with Pandas I#
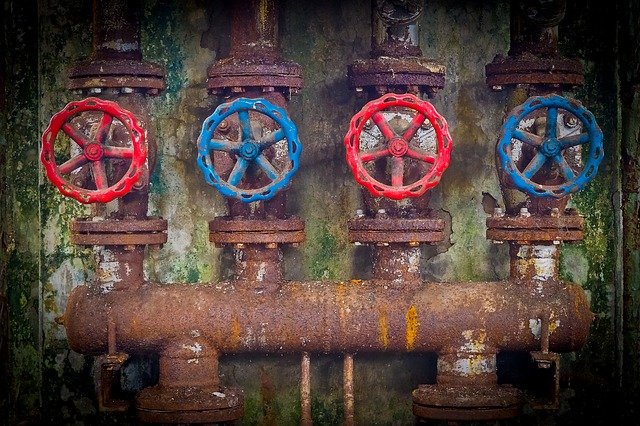
Outline#
In this lecture we will talk about:
How to do Data Analysis
Installing and Using Pandas
Data Manipulation with Pandas
Introducing DataFrames
Creating a DataFrame
Operating on Data in Pandas
How to do Data Analysis#
Here are some common steps of an analysis pipeline (the order isn’t set, and not all elements are necessary):
Load Data
Check file types and encodings.
Check delimiters (space, comma, tab).
Skip rows and columns as needed.
Clean Data
Remove columns not being used.
Deal with “incorrect” data.
Deal with missing data.
Process Data
Create any new columns needed that are combinations or aggregates of other columns (examples include weighted averages, categorizations, groups, etc…).
Find and replace operations (examples inlcude replacing the string ‘Strongly Agree’ with the number 5).
Other substitutions as needed.
Deal with outliers.
Wrangle Data
Restructure data format (columns and rows).
Merge other data sources into your dataset.
Exploratory Data Analysis
More about thisafter the last class of the week
Data Analysis (not required until Milestone 3).
In this course we will some data analysis, but the possibilities are endless here!
Export reports/data analyses and visualizations.
Data Manipulation with Pandas#
Attribution#
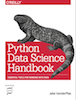
This notebook contains an excerpt from the Python Data Science Handbook by Jake VanderPlas; the content is available on GitHub.
The text is released under the CC-BY-NC-ND license, and code is released under the MIT license. If you find this content useful, please consider supporting the work by buying the book!
In this chapter, we will be looking in detail at the data structures provided by the Pandas library.
Pandas is a newer package built on top of NumPy, and provides an efficient implementation of a DataFrame
.
DataFrame
s are essentially multidimensional arrays with attached row and column labels, and often with heterogeneous types and/or missing data.
As well as offering a convenient storage interface for labeled data, Pandas implements a number of powerful data operations familiar to users of both database frameworks and spreadsheet programs.
Pandas, and in particular its DataFrame
object, builds on the NumPy array structure and provides efficient access to these sorts of “data munging” tasks that occupy much of a data scientist’s time.
In this chapter, we will focus on the mechanics of using the DataFrame
and related structures effectively.
We will use examples drawn from real datasets where appropriate, but these examples are not necessarily the focus.
Installing and Using Pandas#
Installation of Pandas on your system requires NumPy to be installed, and if building the library from source, requires the appropriate tools to compile the C and Cython sources on which Pandas is built. Details on this installation can be found in the Pandas documentation.
To install pandas
, open up a Terminal and install it using conda:
conda install -c conda-forge pandas
Just as we generally import NumPy under the alias np
, we will import Pandas under the alias pd
:
import numpy as np
import pandas as pd
This import convention will be used throughout the remainder of this book.
Once Pandas is installed, and imported you can check the version:
pd.__version__
'2.1.4'
Reminder about Built-In Documentation#
As you read through this chapter, don’t forget that IPython gives you the ability to quickly explore the contents of a package (by using the tab-completion feature) as well as the documentation of various functions (using the ?
character). (Refer back to Help and Documentation in IPython if you need a refresher on this.)
For example, to display all the contents of the pandas namespace, you can type
In [3]: pd.<TAB>
And to display Pandas’s built-in documentation, you can use this:
In [4]: pd?
More detailed documentation, along with tutorials and other resources, can be found at http://pandas.pydata.org/.
pd.read_csv?
Introducing DataFrames#
At the very basic level, Pandas objects can be thought of as enhanced versions of NumPy structured arrays in which the rows and columns are identified with labels rather than simple integer indices.
As we will see during the course of this chapter, Pandas provides a host of useful tools, methods, and functionality on top of the basic data structures, but nearly everything that follows will require an understanding of what these structures are.
Thus, before we go any further, let’s introduce these three fundamental Pandas data structures: the Series
, DataFrame
, and Index
.
We will start our code sessions with the standard NumPy and Pandas imports:
import numpy as np
import pandas as pd
Creating a DataFrame#
There are several ways of creating DataFrames in Python using Pandas and though it can end up with the same end product, the method of creating them can depend on the format of your data.
Let’s look at a few examples:
DataFrame from URL#
If your dataset exists on the web as a publicly accessible file, you can create a DataFrame directly from the URL to the CSV.
read_csv(path)
is a function from the Pandas package that creates a DataFrame from a CSV file.The argument
path
can be a URL or a reference to a local file.
pd.read_csv("https://github.com/firasm/bits/raw/master/fruits.csv")
Fruit Name | Mass(g) | Colour | Rating | |
---|---|---|---|---|
0 | Apple | 200 | Red | 8 |
1 | Banana | 250 | Yellow | 9 |
2 | Cantoloupe | 600 | Orange | 10 |
3 | Cranberry | 50 | Red | 6 |
4 | Blueberry | 20 | Blue | 9 |
5 | Strawberry | 120 | Red | 10 |
6 | Papaya | 220 | Green | 8 |
7 | Lemon | 200 | Yellow | 9 |
8 | Avocado | 300 | Green | 7 |
9 | Jackfruit | 500 | Yellow | 8 |
I can store the dataframe as an object like this:
fruits = pd.read_csv("https://github.com/firasm/bits/raw/master/fruits.csv")
fruits
Fruit Name | Mass(g) | Colour | Rating | |
---|---|---|---|---|
0 | Apple | 200 | Red | 8 |
1 | Banana | 250 | Yellow | 9 |
2 | Cantoloupe | 600 | Orange | 10 |
3 | Cranberry | 50 | Red | 6 |
4 | Blueberry | 20 | Blue | 9 |
5 | Strawberry | 120 | Red | 10 |
6 | Papaya | 220 | Green | 8 |
7 | Lemon | 200 | Yellow | 9 |
8 | Avocado | 300 | Green | 7 |
9 | Jackfruit | 500 | Yellow | 8 |
You can print the first 5 lines of the dataset using the head()
function (this data set only has 3 lines):
fruits.head()
Fruit Name | Mass(g) | Colour | Rating | |
---|---|---|---|---|
0 | Apple | 200 | Red | 8 |
1 | Banana | 250 | Yellow | 9 |
2 | Cantoloupe | 600 | Orange | 10 |
3 | Cranberry | 50 | Red | 6 |
4 | Blueberry | 20 | Blue | 9 |
fruits["Colour"]
0 Red
1 Yellow
2 Orange
3 Red
4 Blue
5 Red
6 Green
7 Yellow
8 Green
9 Yellow
Name: Colour, dtype: object
fruits["Rating"]
0 8
1 9
2 10
3 6
4 9
5 10
6 8
7 9
8 7
9 8
Name: Rating, dtype: int64
fruits["Fruit Name"]
0 Apple
1 Banana
2 Cantoloupe
3 Cranberry
4 Blueberry
5 Strawberry
6 Papaya
7 Lemon
8 Avocado
9 Jackfruit
Name: Fruit Name, dtype: object
fruits["Rating"].sum()
84
DataFrame from List of Dictionaries#
fruit1 = {"Fruit Name": "Apple", "Mass(g)": 200, "Colour": "Red", "Rating": 8}
fruit2 = {"Fruit Name": "Banana", "Mass(g)": 250, "Colour": "Yellow", "Rating": 9}
fruit3 = {"Fruit Name": "Cantoloupe", "Mass(g)": 600, "Colour": "Orange", "Rating": 10}
pd.DataFrame([fruit1, fruit2, fruit3])
Fruit Name | Mass(g) | Colour | Rating | |
---|---|---|---|---|
0 | Apple | 200 | Red | 8 |
1 | Banana | 250 | Yellow | 9 |
2 | Cantoloupe | 600 | Orange | 10 |
DataFrame from List of Tuples#
fruit_tuples = [
("Apple", 200,"Red", 8),
("Banana", 250, "Yellow", 9),
("Cantoloupe", 600, "Orange", 10),
]
labels = ["Fruit Name", "Mass(g)", "Color", "Rating"]
pd.DataFrame(fruit_tuples, columns=labels)
Fruit Name | Mass(g) | Color | Rating | |
---|---|---|---|---|
0 | Apple | 200 | Red | 8 |
1 | Banana | 250 | Yellow | 9 |
2 | Cantoloupe | 600 | Orange | 10 |
DataFrame from a Dictionary#
fruit_dict = {
"Fruit Name": {0: "Apple", 1: "Banana", 2: "Cantoloupe"},
"Mass(g)": {0: 200, 1: 250, 2: 600},
"Colour": {0: "Red", 1: "Yellow", 2: "Orange"},
"Rating": {0: 8, 1: 9, 2: 10},
}
pd.DataFrame.from_dict(fruit_dict)
Fruit Name | Mass(g) | Colour | Rating | |
---|---|---|---|---|
0 | Apple | 200 | Red | 8 |
1 | Banana | 250 | Yellow | 9 |
2 | Cantoloupe | 600 | Orange | 10 |
Here’s a great cheat sheet from “Practical Business Python (PBP)”:
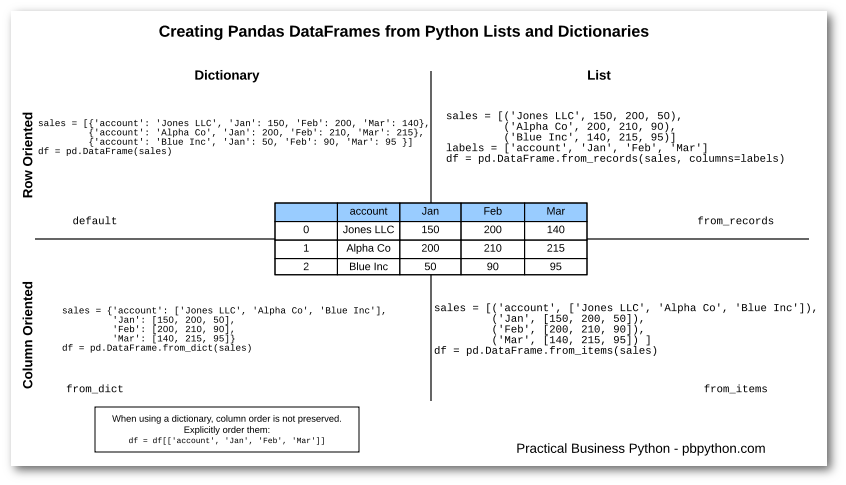
Additionally, the DataFrame
has a columns
attribute, which is an Index
object holding the column labels:
fruits.columns.tolist()
col_names = list(fruits.columns)
col_names[1],col_names[3]
('Mass(g)', 'Rating')
Thus the DataFrame
can be thought of as a generalization of a two-dimensional NumPy array, where both the rows and columns have a generalized index for accessing the data.
Other ways of creating a DataFrame#
From a list of dicts#
Any list of dictionaries can be made into a DataFrame
.
We’ll use a simple list comprehension to create some data:
data = [{"a": i, "b": 2 * i} for i in range(3)] # list comprehension!
pd.DataFrame(data)
a | b | |
---|---|---|
0 | 0 | 0 |
1 | 1 | 2 |
2 | 2 | 4 |
Even if some keys in the dictionary are missing, Pandas will fill them in with NaN
(i.e., “not a number”) values:
pd.DataFrame([{"a": 1, "b": 2}, {"b": 3, "c": 4}])
a | b | c | |
---|---|---|---|
0 | 1.0 | 2 | NaN |
1 | NaN | 3 | 4.0 |
From a two-dimensional NumPy array#
Given a two-dimensional array of data, we can create a DataFrame
with any specified column and index names.
If omitted, an integer index will be used for each:
pd.DataFrame(
np.random.rand(3, 2), columns=["foo", "bar"], index=["Jack", "Ali", "Nusrat"]
)
foo | bar | |
---|---|---|
Jack | 0.759961 | 0.642858 |
Ali | 0.914824 | 0.024106 |
Nusrat | 0.675316 | 0.805373 |
From a NumPy structured array#
We covered structured arrays in Structured Data: NumPy’s Structured Arrays.
A Pandas DataFrame
operates much like a structured array, and can be created directly from one:
A = np.zeros(3, dtype=[("A", "i8"), ("B", "f8")])
A
array([(0, 0.), (0, 0.), (0, 0.)], dtype=[('A', '<i8'), ('B', '<f8')])
pd.DataFrame(A)
A | B | |
---|---|---|
0 | 0 | 0.0 |
1 | 0 | 0.0 |
2 | 0 | 0.0 |
How to Access elements in a DataFrame#
Similarly, we can also think of a DataFrame
as a specialization of a dictionary.
Where a dictionary maps a key to a value, a DataFrame
maps a column name to a Series
of column data.
For example, asking for the 'Mass(g)'
attribute returns a Series
object:
fruits
Fruit Name | Mass(g) | Colour | Rating | |
---|---|---|---|---|
0 | Apple | 200 | Red | 8 |
1 | Banana | 250 | Yellow | 9 |
2 | Cantoloupe | 600 | Orange | 10 |
3 | Cranberry | 50 | Red | 6 |
4 | Blueberry | 20 | Blue | 9 |
5 | Strawberry | 120 | Red | 10 |
6 | Papaya | 220 | Green | 8 |
7 | Lemon | 200 | Yellow | 9 |
8 | Avocado | 300 | Green | 7 |
9 | Jackfruit | 500 | Yellow | 8 |
fruits["Mass(g)"]
0 200
1 250
2 600
3 50
4 20
5 120
6 220
7 200
8 300
9 500
Name: Mass(g), dtype: int64
fruits.loc[0, "Mass(g)"]
200
fruits["Fruit Name"] == "Banana"
0 False
1 True
2 False
3 False
4 False
5 False
6 False
7 False
8 False
9 False
Name: Fruit Name, dtype: bool
fruits[fruits["Fruit Name"] == "Banana"]
Fruit Name | Mass(g) | Colour | Rating | |
---|---|---|---|---|
1 | Banana | 250 | Yellow | 9 |
fruits[fruits["Fruit Name"] == "Banana"]["Colour"]
1 Yellow
Name: Colour, dtype: object
For a DataFrame
, data['col0']
will return the first column.
Because of this, it is probably better to think about DataFrame
s as generalized dictionaries rather than generalized arrays, though both ways of looking at the situation can be useful.
We’ll explore more flexible means of indexing DataFrame
s in Data Indexing and Selection.
Operating on Data in Pandas#
This is where the magic of Pandas really starts to shine - adding, and processing your dataset.
Be back in 5 minutes at 1:05!
Summary Statistics on a Pandas DataFrame#
fruits
Fruit Name | Mass(g) | Colour | Rating | |
---|---|---|---|---|
0 | Apple | 200 | Red | 8 |
1 | Banana | 250 | Yellow | 9 |
2 | Cantoloupe | 600 | Orange | 10 |
3 | Cranberry | 50 | Red | 6 |
4 | Blueberry | 20 | Blue | 9 |
5 | Strawberry | 120 | Red | 10 |
6 | Papaya | 220 | Green | 8 |
7 | Lemon | 200 | Yellow | 9 |
8 | Avocado | 300 | Green | 7 |
9 | Jackfruit | 500 | Yellow | 8 |
fruits.describe()
Mass(g) | Rating | |
---|---|---|
count | 10.000000 | 10.000000 |
mean | 246.000000 | 8.400000 |
std | 183.496897 | 1.264911 |
min | 20.000000 | 6.000000 |
25% | 140.000000 | 8.000000 |
50% | 210.000000 | 8.500000 |
75% | 287.500000 | 9.000000 |
max | 600.000000 | 10.000000 |
You can compute other stats of numeric columns#
fruits.select_dtypes(include="number").mean()
Mass(g) 246.0
Rating 8.4
dtype: float64
fruits.select_dtypes(include="number").median()
Mass(g) 210.0
Rating 8.5
dtype: float64
fruits.select_dtypes(include="number").mode()
Mass(g) | Rating | |
---|---|---|
0 | 200.0 | 8 |
1 | NaN | 9 |
Create a calculated column (from one other column)#
Let’s say we want to create a new column in our fruits DataFrame that is a calculated column.
First let’s print the DataFrame:
fruits
Fruit Name | Mass(g) | Colour | Rating | |
---|---|---|---|---|
0 | Apple | 200 | Red | 8 |
1 | Banana | 250 | Yellow | 9 |
2 | Cantoloupe | 600 | Orange | 10 |
3 | Cranberry | 50 | Red | 6 |
4 | Blueberry | 20 | Blue | 9 |
5 | Strawberry | 120 | Red | 10 |
6 | Papaya | 220 | Green | 8 |
7 | Lemon | 200 | Yellow | 9 |
8 | Avocado | 300 | Green | 7 |
9 | Jackfruit | 500 | Yellow | 8 |
Now, let’s add a column called score
which takes the rating and multiplies it by 50.
We can actually do all of this in just one line:
fruits["Score"] = fruits["Rating"] * 50
fruits
Fruit Name | Mass(g) | Colour | Rating | Score | |
---|---|---|---|---|---|
0 | Apple | 200 | Red | 8 | 400 |
1 | Banana | 250 | Yellow | 9 | 450 |
2 | Cantoloupe | 600 | Orange | 10 | 500 |
3 | Cranberry | 50 | Red | 6 | 300 |
4 | Blueberry | 20 | Blue | 9 | 450 |
5 | Strawberry | 120 | Red | 10 | 500 |
6 | Papaya | 220 | Green | 8 | 400 |
7 | Lemon | 200 | Yellow | 9 | 450 |
8 | Avocado | 300 | Green | 7 | 350 |
9 | Jackfruit | 500 | Yellow | 8 | 400 |
Create a calculated column (from multiple other columns)#
fruits["Score2"] = fruits["Score"] * 50 + fruits["Mass(g)"] * 0.25
fruits
Fruit Name | Mass(g) | Colour | Rating | Score | Score2 | |
---|---|---|---|---|---|---|
0 | Apple | 200 | Red | 8 | 400 | 20050.0 |
1 | Banana | 250 | Yellow | 9 | 450 | 22562.5 |
2 | Cantoloupe | 600 | Orange | 10 | 500 | 25150.0 |
3 | Cranberry | 50 | Red | 6 | 300 | 15012.5 |
4 | Blueberry | 20 | Blue | 9 | 450 | 22505.0 |
5 | Strawberry | 120 | Red | 10 | 500 | 25030.0 |
6 | Papaya | 220 | Green | 8 | 400 | 20055.0 |
7 | Lemon | 200 | Yellow | 9 | 450 | 22550.0 |
8 | Avocado | 300 | Green | 7 | 350 | 17575.0 |
9 | Jackfruit | 500 | Yellow | 8 | 400 | 20125.0 |
Create and apply a custom function to your DataFrame#
Let’s say we wanted to do a complex custom operation on our DataFrame. We will learn how to write Python functions later in the course
def keep_or_discard(x):
"""Decides whether to keep or discard a piece of fruit.
Takes in a Pandas row, and returns either 'keep' or 'discard' depending on
the criteria.
"""
if (x["Mass(g)"] > 220) and (x["Rating"] > 5):
return "keep"
else:
return "discard"
fruits.apply(keep_or_discard, axis="columns")
0 discard
1 keep
2 keep
3 discard
4 discard
5 discard
6 discard
7 discard
8 keep
9 keep
dtype: object
We can now assign the result of this to a new column in our DataFrame.
fruits["Status"] = fruits.apply(keep_or_discard, axis="columns")
fruits
Fruit Name | Mass(g) | Colour | Rating | Score | Score2 | Status | |
---|---|---|---|---|---|---|---|
0 | Apple | 200 | Red | 8 | 400 | 20050.0 | discard |
1 | Banana | 250 | Yellow | 9 | 450 | 22562.5 | keep |
2 | Cantoloupe | 600 | Orange | 10 | 500 | 25150.0 | keep |
3 | Cranberry | 50 | Red | 6 | 300 | 15012.5 | discard |
4 | Blueberry | 20 | Blue | 9 | 450 | 22505.0 | discard |
5 | Strawberry | 120 | Red | 10 | 500 | 25030.0 | discard |
6 | Papaya | 220 | Green | 8 | 400 | 20055.0 | discard |
7 | Lemon | 200 | Yellow | 9 | 450 | 22550.0 | discard |
8 | Avocado | 300 | Green | 7 | 350 | 17575.0 | keep |
9 | Jackfruit | 500 | Yellow | 8 | 400 | 20125.0 | keep |
fruits[ fruits["Status"] == "keep"].reset_index(drop=True)
Fruit Name | Mass(g) | Colour | Rating | Score | Score2 | Status | |
---|---|---|---|---|---|---|---|
0 | Banana | 250 | Yellow | 9 | 450 | 22562.5 | keep |
1 | Cantoloupe | 600 | Orange | 10 | 500 | 25150.0 | keep |
2 | Avocado | 300 | Green | 7 | 350 | 17575.0 | keep |
3 | Jackfruit | 500 | Yellow | 8 | 400 | 20125.0 | keep |
One important feature that we haven’t yet talked about is the “axis” parameter.
axis='rows'
, oraxis=0
, means apply this operation “row-wise”.axis='columns'
, oraxis=1
, means apply this operation “column-wise”.
How to export data#
fruits.to_csv("fruits_processed.csv", index=None)
pd.read_csv("fruits_processed.csv")
Fruit Name | Mass(g) | Colour | Rating | Score | Score2 | Status | |
---|---|---|---|---|---|---|---|
0 | Apple | 200 | Red | 8 | 400 | 20050.0 | discard |
1 | Banana | 250 | Yellow | 9 | 450 | 22562.5 | keep |
2 | Cantoloupe | 600 | Orange | 10 | 500 | 25150.0 | keep |
3 | Cranberry | 50 | Red | 6 | 300 | 15012.5 | discard |
4 | Blueberry | 20 | Blue | 9 | 450 | 22505.0 | discard |
5 | Strawberry | 120 | Red | 10 | 500 | 25030.0 | discard |
6 | Papaya | 220 | Green | 8 | 400 | 20055.0 | discard |
7 | Lemon | 200 | Yellow | 9 | 450 | 22550.0 | discard |
8 | Avocado | 300 | Green | 7 | 350 | 17575.0 | keep |
9 | Jackfruit | 500 | Yellow | 8 | 400 | 20125.0 | keep |
I think this amount of functionality should keep you busy for a while!
More next time!