Class 3C - Data Wrangling with Pandas III¶
We will begin at 12:00 PM! Until then, feel free to use the chat to socialize, and enjoy the music!
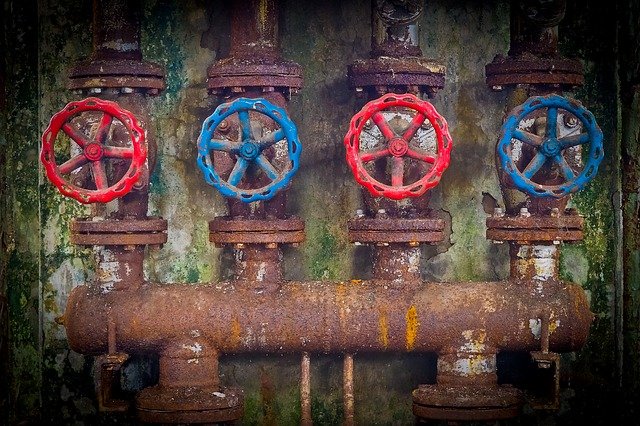
Firas Moosvi
Class Outline¶
In this Class we will talk more about:
Feedback Results
Lab 2 Optional Question
Miscellaneous stuff for Lab 3
Generating Random numbers
Exploring numpy documentation
Aggregation and Grouping
Lab 2 Optional Question¶
3.3: Create a Python program that takes a string text and calculates the frequency of each character (6 marks)¶
text = “””Elephants are mammals of the family Elephantidae and the largest existing land animals. Three species are currently recognised: the African bush elephant, the African forest elephant, and the Asian elephant. Elephantidae is the only surviving family of the order Proboscidea; extinct members include the mastodons. The family Elephantidae also contains several now-extinct groups, including the mammoths and straight-tusked elephants. African elephants have larger ears and concave backs, whereas Asian elephants have smaller ears, and convex or level backs. Distinctive features of all elephants include a long trunk, tusks, large ear flaps, massive legs, and tough but sensitive skin. The trunk, also called a proboscis, is used for breathing, bringing food and water to the mouth, and grasping objects. Tusks, which are derived from the incisor teeth, serve both as weapons and as tools for moving objects and digging. The large ear flaps assist in maintaining a constant body temperature as well as in communication. The pillar-like legs carry their great weight. Elephants are scattered throughout sub-Saharan Africa, South Asia, and Southeast Asia and are found in different habitats, including savannahs, forests, deserts, and marshes. They are herbivorous, and they stay near water when it is accessible. They are considered to be keystone species, due to their impact on their environments.[1] Other animals tend to keep their distance from elephants; the exception is their predators such as lions, tigers, hyenas, and wild dogs, which usually target only young elephants (calves). Elephants have a fission–fusion society, in which multiple family groups come together to socialise. Females (cows) tend to live in family groups, which can consist of one female with her calves or several related females with offspring. The groups, which do not include bulls, are led by the (usually) oldest cow, known as the matriarch.”””
Hint: There are many ways to do this, the most “elegant” way uses a mixture of sets, and the Counter
modules from collections
Sample output:¶
‘ ‘: Count of 293 and Percentage of 15.2%
‘(‘: Count of 3 and Percentage of 0.2%
‘)’: Count of 3 and Percentage of 0.2%
‘,’: Count of 32 and Percentage of 1.7%
‘-‘: Count of 4 and Percentage of 0.2%
‘.’: Count of 17 and Percentage of 0.9%
‘1’: Count of 1 and Percentage of 0.1%
‘:’: Count of 1 and Percentage of 0.1%
‘;’: Count of 2 and Percentage of 0.1%
‘A’: Count of 8 and Percentage of 0.4%
‘D’: Count of 1 and Percentage of 0.1%
‘E’: Count of 6 and Percentage of 0.3%
‘F’: Count of 1 and Percentage of 0.1%
‘O’: Count of 1 and Percentage of 0.1%
‘P’: Count of 1 and Percentage of 0.1%
‘S’: Count of 3 and Percentage of 0.2%
‘T’: Count of 9 and Percentage of 0.5%
‘[‘: Count of 1 and Percentage of 0.1%
‘]’: Count of 1 and Percentage of 0.1%
‘a’: Count of 147 and Percentage of 7.6%
‘b’: Count of 20 and Percentage of 1.0%
‘c’: Count of 55 and Percentage of 2.8%
‘d’: Count of 53 and Percentage of 2.7%
‘e’: Count of 189 and Percentage of 9.8%
‘f’: Count of 32 and Percentage of 1.7%
‘g’: Count of 37 and Percentage of 1.9%
‘h’: Count of 84 and Percentage of 4.4%
‘i’: Count of 109 and Percentage of 5.6%
‘j’: Count of 2 and Percentage of 0.1%
‘k’: Count of 12 and Percentage of 0.6%
‘l’: Count of 80 and Percentage of 4.1%
‘m’: Count of 35 and Percentage of 1.8%
‘n’: Count of 114 and Percentage of 5.9%
‘o’: Count of 87 and Percentage of 4.5%
‘p’: Count of 34 and Percentage of 1.8%
‘r’: Count of 92 and Percentage of 4.8%
‘s’: Count of 131 and Percentage of 6.8%
‘t’: Count of 120 and Percentage of 6.2%
‘u’: Count of 41 and Percentage of 2.1%
‘v’: Count of 22 and Percentage of 1.1%
‘w’: Count of 19 and Percentage of 1.0%
‘x’: Count of 5 and Percentage of 0.3%
‘y’: Count of 21 and Percentage of 1.1%
‘–’: Count of 1 and Percentage of 0.1%
Hint: There are many ways to do this, the most “elegant” way uses a mixture of sets, and the Counter modules from collections
text = """Elephants are mammals of the family Elephantidae and the largest existing land animals. Three species are currently recognised: the African bush elephant, the African forest elephant, and the Asian elephant. Elephantidae is the only surviving family of the order Proboscidea; extinct members include the mastodons. The family Elephantidae also contains several now-extinct groups, including the mammoths and straight-tusked elephants. African elephants have larger ears and concave backs, whereas Asian elephants have smaller ears, and convex or level backs. Distinctive features of all elephants include a long trunk, tusks, large ear flaps, massive legs, and tough but sensitive skin. The trunk, also called a proboscis, is used for breathing, bringing food and water to the mouth, and grasping objects. Tusks, which are derived from the incisor teeth, serve both as weapons and as tools for moving objects and digging. The large ear flaps assist in maintaining a constant body temperature as well as in communication. The pillar-like legs carry their great weight. Elephants are scattered throughout sub-Saharan Africa, South Asia, and Southeast Asia and are found in different habitats, including savannahs, forests, deserts, and marshes. They are herbivorous, and they stay near water when it is accessible. They are considered to be keystone species, due to their impact on their environments.[1] Other animals tend to keep their distance from elephants; the exception is their predators such as lions, tigers, hyenas, and wild dogs, which usually target only young elephants (calves). Elephants have a fission–fusion society, in which multiple family groups come together to socialise. Females (cows) tend to live in family groups, which can consist of one female with her calves or several related females with offspring. The groups, which do not include bulls, are led by the (usually) oldest cow, known as the matriarch."""
letters = list(set(text.lower())) # I think it makes more sense to combine the capital and the lower-case letters
len(letters)
36
text_length = len(text)
for l in sorted(letters):
lcount = text.lower().count(l)
print(f"For the character '{l}': Count of {lcount} and Percentage of {100*lcount/text_length:.1f} %")
# this solution was inspired by this SO post: https://stackoverflow.com/a/1155648
For the character ' ': Count of 293 and Percentage of 15.2 %
For the character '(': Count of 3 and Percentage of 0.2 %
For the character ')': Count of 3 and Percentage of 0.2 %
For the character ',': Count of 32 and Percentage of 1.7 %
For the character '-': Count of 4 and Percentage of 0.2 %
For the character '.': Count of 17 and Percentage of 0.9 %
For the character '1': Count of 1 and Percentage of 0.1 %
For the character ':': Count of 1 and Percentage of 0.1 %
For the character ';': Count of 2 and Percentage of 0.1 %
For the character '[': Count of 1 and Percentage of 0.1 %
For the character ']': Count of 1 and Percentage of 0.1 %
For the character 'a': Count of 155 and Percentage of 8.0 %
For the character 'b': Count of 20 and Percentage of 1.0 %
For the character 'c': Count of 55 and Percentage of 2.8 %
For the character 'd': Count of 54 and Percentage of 2.8 %
For the character 'e': Count of 195 and Percentage of 10.1 %
For the character 'f': Count of 33 and Percentage of 1.7 %
For the character 'g': Count of 37 and Percentage of 1.9 %
For the character 'h': Count of 84 and Percentage of 4.4 %
For the character 'i': Count of 109 and Percentage of 5.6 %
For the character 'j': Count of 2 and Percentage of 0.1 %
For the character 'k': Count of 12 and Percentage of 0.6 %
For the character 'l': Count of 80 and Percentage of 4.1 %
For the character 'm': Count of 35 and Percentage of 1.8 %
For the character 'n': Count of 114 and Percentage of 5.9 %
For the character 'o': Count of 88 and Percentage of 4.6 %
For the character 'p': Count of 35 and Percentage of 1.8 %
For the character 'r': Count of 92 and Percentage of 4.8 %
For the character 's': Count of 134 and Percentage of 6.9 %
For the character 't': Count of 129 and Percentage of 6.7 %
For the character 'u': Count of 41 and Percentage of 2.1 %
For the character 'v': Count of 22 and Percentage of 1.1 %
For the character 'w': Count of 19 and Percentage of 1.0 %
For the character 'x': Count of 5 and Percentage of 0.3 %
For the character 'y': Count of 21 and Percentage of 1.1 %
For the character '–': Count of 1 and Percentage of 0.1 %
Miscellaneous stuff from Lab 3¶
Generating Random numbers
Exploring numpy documentation
import random
import numpy as np
# for loop method
rints= []
for i in range(10):
rints.append(random.randrange(5,50))
# list comprehension method
[random.randrange(5,50) for i in range(10)]
[11, 27, 25, 14, 11, 40, 14, 24, 17, 44]
# another way
np.random.rand(10)
array([0.09606352, 0.45121143, 0.87241139, 0.47308334, 0.09519842,
0.73981544, 0.09593441, 0.36333275, 0.32305733, 0.48241197])
# another way
rints2 = np.arange(5,50)
for i in range(10):
print(random.choice(rints2))
38
8
21
5
6
40
21
34
26
35
random.choice(rints)
47
Aggregation and Grouping¶
Attribution¶
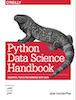
This notebook contains an excerpt from the Python Data Science Handbook by Jake VanderPlas; the content is available on GitHub.
The text is released under the CC-BY-NC-ND license, and code is released under the MIT license. If you find this content useful, please consider supporting the work by buying the book!
An essential piece of analysis of large data is efficient summarization: computing aggregations like sum()
, mean()
, median()
, min()
, and max()
, in which a single number gives insight into the nature of a potentially large dataset.
In this section, we’ll explore aggregations in Pandas, from simple operations akin to what we’ve seen on NumPy arrays, to more sophisticated operations based on the concept of a groupby
.
For convenience, we’ll use the same display
magic function that we’ve seen in previous sections:
import numpy as np
import pandas as pd
class display(object):
"""Display HTML representation of multiple objects"""
template = """<div style="float: left; padding: 10px;">
<p style='font-family:"Courier New", Courier, monospace'>{0}</p>{1}
</div>"""
def __init__(self, *args):
self.args = args
def _repr_html_(self):
return '\n'.join(self.template.format(a, eval(a)._repr_html_())
for a in self.args)
def __repr__(self):
return '\n\n'.join(a + '\n' + repr(eval(a))
for a in self.args)
Planets Data¶
Here we will use the Planets dataset, available via the Seaborn package (see Visualization With Seaborn). It gives information on planets that astronomers have discovered around other stars (known as extrasolar planets or exoplanets for short). It can be downloaded with a simple Seaborn command:
import seaborn as sns # `conda install seaborn` in your terminal
planets = sns.load_dataset('planets')
planets
method | number | orbital_period | mass | distance | year | |
---|---|---|---|---|---|---|
0 | Radial Velocity | 1 | 269.300000 | 7.10 | 77.40 | 2006 |
1 | Radial Velocity | 1 | 874.774000 | 2.21 | 56.95 | 2008 |
2 | Radial Velocity | 1 | 763.000000 | 2.60 | 19.84 | 2011 |
3 | Radial Velocity | 1 | 326.030000 | 19.40 | 110.62 | 2007 |
4 | Radial Velocity | 1 | 516.220000 | 10.50 | 119.47 | 2009 |
... | ... | ... | ... | ... | ... | ... |
1030 | Transit | 1 | 3.941507 | NaN | 172.00 | 2006 |
1031 | Transit | 1 | 2.615864 | NaN | 148.00 | 2007 |
1032 | Transit | 1 | 3.191524 | NaN | 174.00 | 2007 |
1033 | Transit | 1 | 4.125083 | NaN | 293.00 | 2008 |
1034 | Transit | 1 | 4.187757 | NaN | 260.00 | 2008 |
1035 rows × 6 columns
planets.head()
method | number | orbital_period | mass | distance | year | |
---|---|---|---|---|---|---|
0 | Radial Velocity | 1 | 269.300 | 7.10 | 77.40 | 2006 |
1 | Radial Velocity | 1 | 874.774 | 2.21 | 56.95 | 2008 |
2 | Radial Velocity | 1 | 763.000 | 2.60 | 19.84 | 2011 |
3 | Radial Velocity | 1 | 326.030 | 19.40 | 110.62 | 2007 |
4 | Radial Velocity | 1 | 516.220 | 10.50 | 119.47 | 2009 |
This has some details on the 1,000+ extrasolar planets discovered up to 2014.
Simple Aggregation in Pandas¶
Earlier, we explored some of the data aggregations available for NumPy arrays (“Aggregations: Min, Max, and Everything In Between”).
As with a one-dimensional NumPy array, for a Pandas Series
the aggregates return a single value:
rng = np.random.RandomState(42)
ser = pd.Series(rng.rand(5))
ser
0 0.374540
1 0.950714
2 0.731994
3 0.598658
4 0.156019
dtype: float64
ser.sum()
2.811925491708157
ser.mean()
0.5623850983416314
For a DataFrame
, by default the aggregates return results within each column:
import pandas as pd
df = pd.DataFrame({'A': rng.rand(5),
'B': rng.rand(5)})
df
A | B | |
---|---|---|
0 | 0.155995 | 0.020584 |
1 | 0.058084 | 0.969910 |
2 | 0.866176 | 0.832443 |
3 | 0.601115 | 0.212339 |
4 | 0.708073 | 0.181825 |
df.mean()
A 0.477888
B 0.443420
dtype: float64
By specifying the axis
argument, you can instead aggregate within each row:
df.mean(axis='columns')
0 0.088290
1 0.513997
2 0.849309
3 0.406727
4 0.444949
dtype: float64
Pandas Series
and DataFrame
s include all of the common aggregates mentioned in Aggregations: Min, Max, and Everything In Between; in addition, there is a convenience method describe()
that computes several common aggregates for each column and returns the result.
Let’s use this on the Planets data, for now dropping rows with missing values:
planets.dropna().describe()
number | orbital_period | mass | distance | year | |
---|---|---|---|---|---|
count | 498.00000 | 498.000000 | 498.000000 | 498.000000 | 498.000000 |
mean | 1.73494 | 835.778671 | 2.509320 | 52.068213 | 2007.377510 |
std | 1.17572 | 1469.128259 | 3.636274 | 46.596041 | 4.167284 |
min | 1.00000 | 1.328300 | 0.003600 | 1.350000 | 1989.000000 |
25% | 1.00000 | 38.272250 | 0.212500 | 24.497500 | 2005.000000 |
50% | 1.00000 | 357.000000 | 1.245000 | 39.940000 | 2009.000000 |
75% | 2.00000 | 999.600000 | 2.867500 | 59.332500 | 2011.000000 |
max | 6.00000 | 17337.500000 | 25.000000 | 354.000000 | 2014.000000 |
This can be a useful way to begin understanding the overall properties of a dataset.
For example, we see in the year
column that although exoplanets were discovered as far back as 1989, half of all known expolanets were not discovered until 2010 or after.
This is largely thanks to the Kepler mission, which is a space-based telescope specifically designed for finding eclipsing planets around other stars.
The following table summarizes some other built-in Pandas aggregations:
Aggregation |
Description |
---|---|
|
Total number of items |
|
First and last item |
|
Mean and median |
|
Minimum and maximum |
|
Standard deviation and variance |
|
Mean absolute deviation |
|
Product of all items |
|
Sum of all items |
These are all methods of DataFrame
and Series
objects.
To go deeper into the data, however, simple aggregates are often not enough.
The next level of data summarization is the groupby
operation, which allows you to quickly and efficiently compute aggregates on subsets of data.
Break - back at 1 PM!¶
GroupBy: Split, Apply, Combine¶
Simple aggregations can give you a flavor of your dataset, but often we would prefer to aggregate conditionally on some label or index: this is implemented in the so-called groupby
operation.
The name “group by” comes from a command in the SQL database language, but it is perhaps more illuminative to think of it in the terms first coined by Hadley Wickham of Rstats fame: split, apply, combine.
Split, apply, combine¶
A canonical example of this split-apply-combine operation, where the “apply” is a summation aggregation, is illustrated in this figure:
This makes clear what the groupby
accomplishes:
The split step involves breaking up and grouping a
DataFrame
depending on the value of the specified key.The apply step involves computing some function, usually an aggregate, transformation, or filtering, within the individual groups.
The combine step merges the results of these operations into an output array.
While this could certainly be done manually using some combination of the masking, aggregation, and merging commands covered earlier, an important realization is that the intermediate splits do not need to be explicitly instantiated. Rather, the GroupBy
can (often) do this in a single pass over the data, updating the sum, mean, count, min, or other aggregate for each group along the way.
The power of the GroupBy
is that it abstracts away these steps: the user need not think about how the computation is done under the hood, but rather thinks about the operation as a whole.
As a concrete example, let’s take a look at using Pandas for the computation shown in this diagram.
We’ll start by creating the input DataFrame
:
df = pd.DataFrame({'key': ['A', 'B', 'C', 'A', 'B', 'C'],
'data': range(6)}, columns=['key', 'data'])
df
key | data | |
---|---|---|
0 | A | 0 |
1 | B | 1 |
2 | C | 2 |
3 | A | 3 |
4 | B | 4 |
5 | C | 5 |
The most basic split-apply-combine operation can be computed with the groupby()
method of DataFrame
s, passing the name of the desired key column:
df.groupby('key')
<pandas.core.groupby.generic.DataFrameGroupBy object at 0x7fdab1254190>
Notice that what is returned is not a set of DataFrame
s, but a DataFrameGroupBy
object.
This object is where the magic is: you can think of it as a special view of the DataFrame
, which is poised to dig into the groups but does no actual computation until the aggregation is applied.
This “lazy evaluation” approach means that common aggregates can be implemented very efficiently in a way that is almost transparent to the user.
To produce a result, we can apply an aggregate to this DataFrameGroupBy
object, which will perform the appropriate apply/combine steps to produce the desired result:
df.groupby('key').sum()
data | |
---|---|
key | |
A | 3 |
B | 5 |
C | 7 |
The sum()
method is just one possibility here; you can apply virtually any common Pandas or NumPy aggregation function, as well as virtually any valid DataFrame
operation, as we will see in the following discussion.
The GroupBy object¶
The GroupBy
object is a very flexible abstraction.
In many ways, you can simply treat it as if it’s a collection of DataFrame
s, and it does the difficult things under the hood. Let’s see some examples using the Planets data.
Perhaps the most important operations made available by a GroupBy
are aggregate, filter, transform, and apply.
We’ll discuss each of these more fully in “Aggregate, Filter, Transform, Apply”, but before that let’s introduce some of the other functionality that can be used with the basic GroupBy
operation.
Column indexing¶
The GroupBy
object supports column indexing in the same way as the DataFrame
, and returns a modified GroupBy
object.
For example:
planets
method | number | orbital_period | mass | distance | year | |
---|---|---|---|---|---|---|
0 | Radial Velocity | 1 | 269.300000 | 7.10 | 77.40 | 2006 |
1 | Radial Velocity | 1 | 874.774000 | 2.21 | 56.95 | 2008 |
2 | Radial Velocity | 1 | 763.000000 | 2.60 | 19.84 | 2011 |
3 | Radial Velocity | 1 | 326.030000 | 19.40 | 110.62 | 2007 |
4 | Radial Velocity | 1 | 516.220000 | 10.50 | 119.47 | 2009 |
... | ... | ... | ... | ... | ... | ... |
1030 | Transit | 1 | 3.941507 | NaN | 172.00 | 2006 |
1031 | Transit | 1 | 2.615864 | NaN | 148.00 | 2007 |
1032 | Transit | 1 | 3.191524 | NaN | 174.00 | 2007 |
1033 | Transit | 1 | 4.125083 | NaN | 293.00 | 2008 |
1034 | Transit | 1 | 4.187757 | NaN | 260.00 | 2008 |
1035 rows × 6 columns
planets.groupby('method')
<pandas.core.groupby.generic.DataFrameGroupBy object at 0x7fdab12542e0>
planets.groupby('method')['orbital_period']
<pandas.core.groupby.generic.SeriesGroupBy object at 0x7fdaf4f8f8e0>
Here we’ve selected a particular Series
group from the original DataFrame
group by reference to its column name.
As with the GroupBy
object, no computation is done until we call some aggregate on the object:
planets.groupby('method')['orbital_period'].median()
method
Astrometry 631.180000
Eclipse Timing Variations 4343.500000
Imaging 27500.000000
Microlensing 3300.000000
Orbital Brightness Modulation 0.342887
Pulsar Timing 66.541900
Pulsation Timing Variations 1170.000000
Radial Velocity 360.200000
Transit 5.714932
Transit Timing Variations 57.011000
Name: orbital_period, dtype: float64
This gives an idea of the general scale of orbital periods (in days) that each method is sensitive to.
Iteration over groups¶
The GroupBy
object supports direct iteration over the groups, returning each group as a Series
or DataFrame
:
for (method, group) in planets.groupby('method'):
print(f"{method:30s} shape={group.shape}")
Astrometry shape=(2, 6)
Eclipse Timing Variations shape=(9, 6)
Imaging shape=(38, 6)
Microlensing shape=(23, 6)
Orbital Brightness Modulation shape=(3, 6)
Pulsar Timing shape=(5, 6)
Pulsation Timing Variations shape=(1, 6)
Radial Velocity shape=(553, 6)
Transit shape=(397, 6)
Transit Timing Variations shape=(4, 6)
This can be useful for doing certain things manually, though it is often much faster to use the built-in apply
functionality, which we will discuss momentarily.
Dispatch methods¶
Through some Python class magic, any method not explicitly implemented by the GroupBy
object will be passed through and called on the groups, whether they are DataFrame
or Series
objects.
For example, you can use the describe()
method of DataFrame
s to perform a set of aggregations that describe each group in the data:
planets.groupby('method')['year'].describe().unstack()
method
count Astrometry 2.0
Eclipse Timing Variations 9.0
Imaging 38.0
Microlensing 23.0
Orbital Brightness Modulation 3.0
...
max Pulsar Timing 2011.0
Pulsation Timing Variations 2007.0
Radial Velocity 2014.0
Transit 2014.0
Transit Timing Variations 2014.0
Length: 80, dtype: float64
Looking at this table helps us to better understand the data: for example, the vast majority of planets have been discovered by the Radial Velocity and Transit methods, though the latter only became common (due to new, more accurate telescopes) in the last decade. The newest methods seem to be Transit Timing Variation and Orbital Brightness Modulation, which were not used to discover a new planet until 2011.
This is just one example of the utility of dispatch methods.
Notice that they are applied to each individual group, and the results are then combined within GroupBy
and returned.
Again, any valid DataFrame
/Series
method can be used on the corresponding GroupBy
object, which allows for some very flexible and powerful operations!
Aggregate, filter, transform, apply¶
The preceding discussion focused on aggregation for the combine operation, but there are more options available.
In particular, GroupBy
objects have aggregate()
, filter()
, transform()
, and apply()
methods that efficiently implement a variety of useful operations before combining the grouped data.
For the purpose of the following subsections, we’ll use this DataFrame
:
rng = np.random.RandomState(0)
df = pd.DataFrame({'key': ['A', 'B', 'C', 'A', 'B', 'C'],
'data1': range(6),
'data2': rng.randint(0, 10, 6)},
columns = ['key', 'data1', 'data2'])
df
key | data1 | data2 | |
---|---|---|---|
0 | A | 0 | 5 |
1 | B | 1 | 0 |
2 | C | 2 | 3 |
3 | A | 3 | 3 |
4 | B | 4 | 7 |
5 | C | 5 | 9 |
Aggregation¶
We’re now familiar with GroupBy
aggregations with sum()
, median()
, and the like, but the aggregate()
method allows for even more flexibility.
It can take a string, a function, or a list thereof, and compute all the aggregates at once.
Here is a quick example combining all these:
df.groupby('key').aggregate(['min', np.median, max])
data1 | data2 | |||||
---|---|---|---|---|---|---|
min | median | max | min | median | max | |
key | ||||||
A | 0 | 1.5 | 3 | 3 | 4.0 | 5 |
B | 1 | 2.5 | 4 | 0 | 3.5 | 7 |
C | 2 | 3.5 | 5 | 3 | 6.0 | 9 |
Another useful pattern is to pass a dictionary mapping column names to operations to be applied on that column:
df.groupby('key').aggregate({'data1': 'min',
'data2': 'max'})
data1 | data2 | |
---|---|---|
key | ||
A | 0 | 5 |
B | 1 | 7 |
C | 2 | 9 |
Filtering¶
A filtering operation allows you to drop data based on the group properties. For example, we might want to keep all groups in which the standard deviation is larger than some critical value:
def filter_func(x):
return x['data2'].std() > 4
display('df', "df.groupby('key').std()", "df.groupby('key').filter(filter_func)")
df
key | data1 | data2 | |
---|---|---|---|
0 | A | 0 | 5 |
1 | B | 1 | 0 |
2 | C | 2 | 3 |
3 | A | 3 | 3 |
4 | B | 4 | 7 |
5 | C | 5 | 9 |
df.groupby('key').std()
data1 | data2 | |
---|---|---|
key | ||
A | 2.12132 | 1.414214 |
B | 2.12132 | 4.949747 |
C | 2.12132 | 4.242641 |
df.groupby('key').filter(filter_func)
key | data1 | data2 | |
---|---|---|---|
1 | B | 1 | 0 |
2 | C | 2 | 3 |
4 | B | 4 | 7 |
5 | C | 5 | 9 |
The filter function should return a Boolean value specifying whether the group passes the filtering. Here because group A does not have a standard deviation greater than 4, it is dropped from the result.
Transformation¶
While aggregation must return a reduced version of the data, transformation can return some transformed version of the full data to recombine. For such a transformation, the output is the same shape as the input. A common example is to center the data by subtracting the group-wise mean:
df.groupby('key').transform(lambda x: x - x.mean())
data1 | data2 | |
---|---|---|
0 | -1.5 | 1.0 |
1 | -1.5 | -3.5 |
2 | -1.5 | -3.0 |
3 | 1.5 | -1.0 |
4 | 1.5 | 3.5 |
5 | 1.5 | 3.0 |
The apply() method¶
The apply()
method lets you apply an arbitrary function to the group results.
The function should take a DataFrame
, and return either a Pandas object (e.g., DataFrame
, Series
) or a scalar; the combine operation will be tailored to the type of output returned.
For example, here is an apply()
that normalizes the first column by the sum of the second:
def norm_by_data2(x):
# x is a DataFrame of group values
x['data1'] /= x['data2'].sum() # "y/= something" is equivalent to "y = y/something"
return x
display('df', "df.groupby('key').apply(norm_by_data2)")
df
key | data1 | data2 | |
---|---|---|---|
0 | A | 0 | 5 |
1 | B | 1 | 0 |
2 | C | 2 | 3 |
3 | A | 3 | 3 |
4 | B | 4 | 7 |
5 | C | 5 | 9 |
df.groupby('key').apply(norm_by_data2)
key | data1 | data2 | |
---|---|---|---|
0 | A | 0.000000 | 5 |
1 | B | 0.142857 | 0 |
2 | C | 0.166667 | 3 |
3 | A | 0.375000 | 3 |
4 | B | 0.571429 | 7 |
5 | C | 0.416667 | 9 |
apply()
within a GroupBy
is quite flexible: the only criterion is that the function takes a DataFrame
and returns a Pandas object or scalar; what you do in the middle is up to you!
Specifying the split key¶
In the simple examples presented before, we split the DataFrame
on a single column name.
This is just one of many options by which the groups can be defined, and we’ll go through some other options for group specification here.
A list, array, series, or index providing the grouping keys¶
The key can be any series or list with a length matching that of the DataFrame
. For example:
L = [0, 1, 0, 1, 2, 0]
display('df', 'df.groupby(L).sum()')
df
key | data1 | data2 | |
---|---|---|---|
0 | A | 0 | 5 |
1 | B | 1 | 0 |
2 | C | 2 | 3 |
3 | A | 3 | 3 |
4 | B | 4 | 7 |
5 | C | 5 | 9 |
df.groupby(L).sum()
data1 | data2 | |
---|---|---|
0 | 7 | 17 |
1 | 4 | 3 |
2 | 4 | 7 |
Of course, this means there’s another, more verbose way of accomplishing the df.groupby('key')
from before:
display('df', "df.groupby(df['key']).sum()")
df
key | data1 | data2 | |
---|---|---|---|
0 | A | 0 | 5 |
1 | B | 1 | 0 |
2 | C | 2 | 3 |
3 | A | 3 | 3 |
4 | B | 4 | 7 |
5 | C | 5 | 9 |
df.groupby(df['key']).sum()
data1 | data2 | |
---|---|---|
key | ||
A | 3 | 8 |
B | 5 | 7 |
C | 7 | 12 |
A dictionary or series mapping index to group¶
Another method is to provide a dictionary that maps index values to the group keys:
df2 = df.set_index('key')
mapping = {'A': 'vowel', 'B': 'consonant', 'C': 'consonant'}
display('df2', 'df2.groupby(mapping).sum()')
df2
data1 | data2 | |
---|---|---|
key | ||
A | 0 | 5 |
B | 1 | 0 |
C | 2 | 3 |
A | 3 | 3 |
B | 4 | 7 |
C | 5 | 9 |
df2.groupby(mapping).sum()
data1 | data2 | |
---|---|---|
key | ||
consonant | 12 | 19 |
vowel | 3 | 8 |
Any Python function¶
Similar to mapping, you can pass any Python function that will input the index value and output the group:
display('df2', 'df2.groupby(str.lower).mean()')
df2
data1 | data2 | |
---|---|---|
key | ||
A | 0 | 5 |
B | 1 | 0 |
C | 2 | 3 |
A | 3 | 3 |
B | 4 | 7 |
C | 5 | 9 |
df2.groupby(str.lower).mean()
data1 | data2 | |
---|---|---|
key | ||
a | 1.5 | 4.0 |
b | 2.5 | 3.5 |
c | 3.5 | 6.0 |
A list of valid keys¶
Further, any of the preceding key choices can be combined to group on a multi-index:
df2.groupby([str.lower, mapping]).mean()
data1 | data2 | ||
---|---|---|---|
key | key | ||
a | vowel | 1.5 | 4.0 |
b | consonant | 2.5 | 3.5 |
c | consonant | 3.5 | 6.0 |
Grouping example¶
As an example of this, in a couple lines of Python code we can put all these together and count discovered planets by method and by decade:
planets
method | number | orbital_period | mass | distance | year | |
---|---|---|---|---|---|---|
0 | Radial Velocity | 1 | 269.300000 | 7.10 | 77.40 | 2006 |
1 | Radial Velocity | 1 | 874.774000 | 2.21 | 56.95 | 2008 |
2 | Radial Velocity | 1 | 763.000000 | 2.60 | 19.84 | 2011 |
3 | Radial Velocity | 1 | 326.030000 | 19.40 | 110.62 | 2007 |
4 | Radial Velocity | 1 | 516.220000 | 10.50 | 119.47 | 2009 |
... | ... | ... | ... | ... | ... | ... |
1030 | Transit | 1 | 3.941507 | NaN | 172.00 | 2006 |
1031 | Transit | 1 | 2.615864 | NaN | 148.00 | 2007 |
1032 | Transit | 1 | 3.191524 | NaN | 174.00 | 2007 |
1033 | Transit | 1 | 4.125083 | NaN | 293.00 | 2008 |
1034 | Transit | 1 | 4.187757 | NaN | 260.00 | 2008 |
1035 rows × 6 columns
decade = 10 * (planets['year'] // 10)
decade
0 2000
1 2000
2 2010
3 2000
4 2000
...
1030 2000
1031 2000
1032 2000
1033 2000
1034 2000
Name: year, Length: 1035, dtype: int64
decade = decade.astype(str) + 's'
decade
0 2000s
1 2000s
2 2010s
3 2000s
4 2000s
...
1030 2000s
1031 2000s
1032 2000s
1033 2000s
1034 2000s
Name: year, Length: 1035, dtype: object
decade.name = 'decade'
planets.groupby(['method', decade])['number'].sum().unstack().fillna(0)
decade | 1980s | 1990s | 2000s | 2010s |
---|---|---|---|---|
method | ||||
Astrometry | 0.0 | 0.0 | 0.0 | 2.0 |
Eclipse Timing Variations | 0.0 | 0.0 | 5.0 | 10.0 |
Imaging | 0.0 | 0.0 | 29.0 | 21.0 |
Microlensing | 0.0 | 0.0 | 12.0 | 15.0 |
Orbital Brightness Modulation | 0.0 | 0.0 | 0.0 | 5.0 |
Pulsar Timing | 0.0 | 9.0 | 1.0 | 1.0 |
Pulsation Timing Variations | 0.0 | 0.0 | 1.0 | 0.0 |
Radial Velocity | 1.0 | 52.0 | 475.0 | 424.0 |
Transit | 0.0 | 0.0 | 64.0 | 712.0 |
Transit Timing Variations | 0.0 | 0.0 | 0.0 | 9.0 |
This shows the power of combining many of the operations we’ve discussed up to this point when looking at realistic datasets. We immediately gain a coarse understanding of when and how planets have been discovered over the past several decades!
Here I would suggest digging into these few lines of code, and evaluating the individual steps to make sure you understand exactly what they are doing to the result. It’s certainly a somewhat complicated example, but understanding these pieces will give you the means to similarly explore your own data.
That’s it!
Have a good weekend!